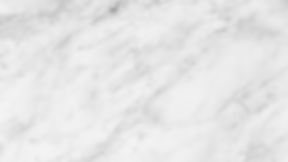
Last updated: 7 - 26 - 2018
CONTROL, OPTIMIZATION, AND ESTIMATION FOR EXERCISE MACHINE
We developed new approaches to estimation, control, and optimization of advanced energy-regenerative cyber-enabled exercise machines (CEEM), which extensively sense or estimate mechanical and biomechanical variables, automatically adjust their own resistance (impedance) to maximize a programmable objective, capable of storing the user energy rather than being dissipated by heat, and provide enhanced information to the user. Specifically, the objective is to maximize activation of target muscle groups. The purpose of enabling such functions in an exercise machine is to optimize machine-based physical conditioning. The control and optimization subsystems of a CEEM must operate on the basis of reliable sensing of key quantities. However, the use of sensors may be undesirable for a variety of reasons, such as invasiveness, cost, noise, hardware complexity, narrow bandwidth, and the possibility of failure. Therefore, we instead used estimation theory with limited sensing of noisy human-machine measurement signals to estimate the key quantities in presence of imperfect system models.
To estimate the human biomechanical signals, we constructed and developed an extended Kalman filter, a sliding mode observer, a high gain observer, and a novel adaptive sliding mode observer for a dual agonist-antagonist muscle system operated with a backstepping controller. The controller is used to control the muscle length and to provide a framework within which to conduct state estimation. We developed a dimensionless agonist–antagonist muscle model based on Hill muscle model, which has advantages such as generalization and extension to any kind of muscle type. We used two noisy measurement signals to estimate the muscle model states: muscle lengths, which were measured from joint angles; and muscle activations, which were measured from EMG sensors. We designed observers to account for different real-life estimation scenarios: estimation with model uncertainties, estimation with perfect knowledge of measurement signals, and estimation with no knowledge of the activation signals that led to the design of unknown inputs observers. The theoretical proofs were provided to show the stability and robustness of the observers. We incorporated muscle constraints using a projection approach to further improve the estimates. The states of the system were estimated very accurately and were converged quickly in spite of significant initialization errors. We extended our work to address the problem of state estimation of a two-joint six-muscle linkage that included the dynamics of both the motion and the muscle. We estimated the joint velocities, tendon-muscle lengths, and rates of tendon muscle lengths using the noisy measurements of the joint angles. The standard deviations of the estimation errors were very small, on the order of 10E-4–10E-2 rad for joint angles, 10E-3–10E-1 rad/s for joint velocities, 10E-4–10E-3 m for muscle-tendon length, and 10E-5–10E-3 m/s for muscle-tendon length rates.
Photo gallery for control and estimation of advanced exercise machine project
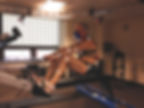
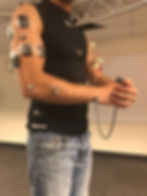
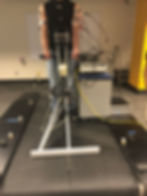
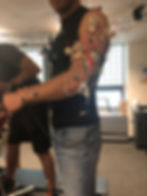
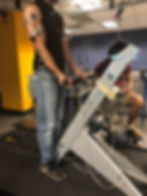
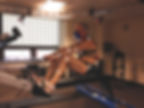
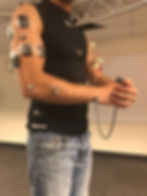
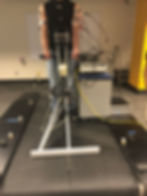
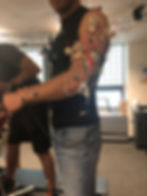
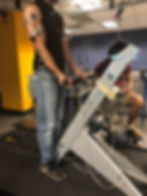
Human trial with a custom-built rowing exercise machine at the Cleveland State University in collaboration with Parker Hannifin Human Motion Control Laboratory
Publications:
(1) H. Mohammadi, D. Simon, and H. Richter, “State Estimation of a Muscle-Driven Linkage,” IEEE American Control Conference, Milwaukee, Wisconsin, June 2018.
(2) H. Mohammadi, G. Khademi, D. Simon, and H. Richter, "State Estimation of an Advanced Rowing Machine Using Optimized Kalman Filtering," in Dynamic Systems and Control Conference (DSCC), Tysons, Virginia, October 2017. DOI: 10.1115/DSCC2017-5319
(3) T. Nguyen, H. Warner, H. Mohammadi, D. Simon, and H. Richter, “On the State Estimation of an Agonistic-Antagonistic Muscle System,” in Dynamic Systems and Control Conference (DSCC), Tysons, Virginia, October 2017.
(4) T. T. Nguyen, H. Warner, H. La, H. Mohammadi, D. Simon, and H. Richter, “State estimation for an agonistic-antagonistic muscle system,” Asian Journal of Control, in print, 2018.
(5) H. Mohammadi, H. Yao, G. Khademi, T. T. Nguyen, D. Simon, and H. Richter, “Extended Kalman Filtering for State Estimation of a Hill Muscle Model,” IET Control Theory and Applications, vol. 12, no. 3, pp. 384-394, 2017.
DOI: 10.1049/iet-cta.2017.0645 (PDF)
Introduction to Advanced Cyber-Enabled Exercise Machine